Understanding Image Annotation Tools for Machine Learning
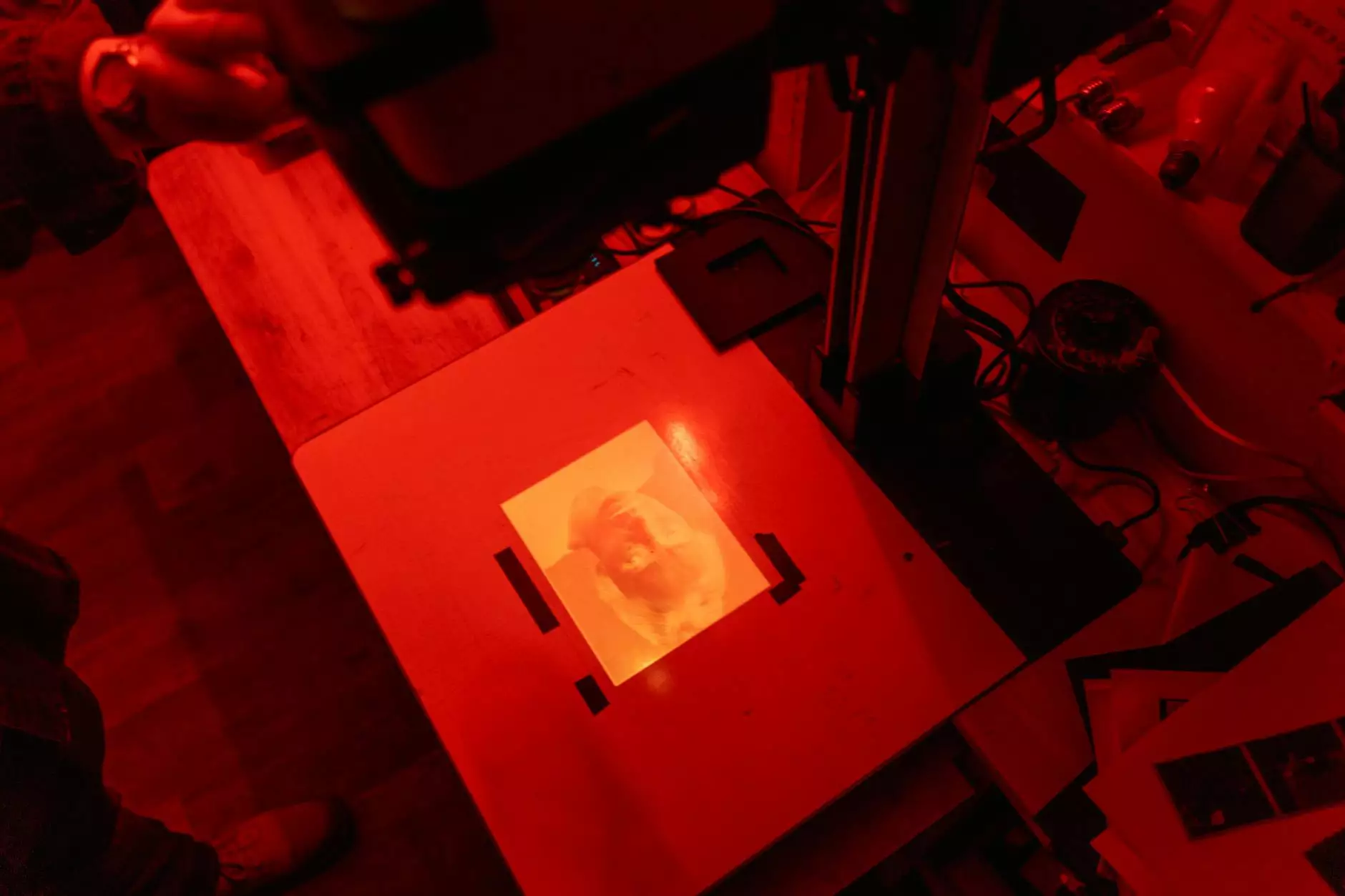
In the rapidly evolving field of machine learning, the importance of accurate and efficient data preparation is paramount. One of the critical steps in this process is image annotation, which involves labeling and tagging images to provide context for algorithms. In this article, we will delve deep into the realm of image annotation tools for machine learning, exploring their significance, process, types, and much more.
The Role of Image Annotation in Machine Learning
Machine learning models require data to learn and make predictions. When it comes to visual data, the machine learning process relies heavily on properly annotated images. Without proper labels, models struggle to understand what they are processing, leading to inaccurate outcomes.
What is Image Annotation?
Image annotation is the process of labeling images within datasets to create informative and structured data. This practice is essential for enabling machine learning algorithms to identify patterns and features within the visual context. Types of annotation include:
- Bounding boxes: Drawing rectangles around objects in images to identify them.
- Segmentation: Dividing images into multiple segments for detailed analysis.
- Key Point Annotation: Marking specific points on an object, crucial in tasks like facial recognition.
- Polygon Annotation: Creating polygon shapes around objects for precise outlining.
- Text Annotation: Adding textual descriptions or tags to images to provide context.
Benefits of Using Image Annotation Tools for Machine Learning
The rise of image annotation tools for machine learning has transformed the data preparation landscape. Here are some benefits these tools offer:
1. Improved Accuracy
Correctly annotated images ensure that machine learning models learn from precise and relevant data. This accuracy is crucial for generating reliable outputs in applications ranging from autonomous vehicles to medical imaging.
2. Efficiency in Data Handling
Manually annotating data can be time-consuming. Image annotation tools streamline this process, allowing for bulk annotations, automated tasks, and a more manageable workflow. This efficiency frees up valuable time and resources.
3. Scalability
As the volume of data increases, so does the necessity for scalable solutions. Image annotation tools are designed to handle large datasets, enabling businesses to scale operations without compromising quality.
4. Collaboration Features
Many modern annotation tools offer collaboration features that allow teams to work together seamlessly. These features facilitate real-time feedback and adjustments, ensuring that projects can adapt quickly to new requirements.
Types of Image Annotation Tools
Numerous image annotation tools exist today, each tailored to specific needs and workflows. Here’s an overview of the varieties:
1. Online Annotation Tools
These are cloud-based platforms allowing users to access annotation features through a web browser. They are typically user-friendly and do not require extensive setup.
2. Desktop Annotation Software
Such tools are installed directly on a computer. They might offer more advanced features and processing capabilities, making them suitable for larger, more complex datasets.
3. Automated Annotation Tools
Harnessing machine learning capabilities, these tools can automate portions of the annotation process, significantly speeding up tasks. While they still require supervision, they are crucial for handling vast amounts of data.
4. Open-source Annotation Tools
Open-source tools are a popular choice due to their flexibility and adaptability. Users can modify the software to suit their specific needs, fostering innovation and collaboration within the developer community.
How to Choose the Right Image Annotation Tool
Selecting the appropriate image annotation tool for machine learning can greatly impact the efficiency and accuracy of your project. Here are key factors to consider:
1. Type of Data
Understand the nature of your images and the complexity of your annotation tasks. Some tools are better suited for specific types of annotations, such as bounding box versus segmentation.
2. User-Friendliness
Look for tools that are intuitive and easy to use, especially for teams with varied levels of technical expertise. A steep learning curve can slow down your project.
3. Collaboration Features
Consider whether the tool supports collaborative efforts. If multiple team members are involved, features like real-time editing and commenting are advantageous.
4. Pricing Model
Evaluate the pricing structures of tools to ensure they align with your budget. Some tools offer free trials, allowing you to assess their usability before making a commitment.
5. Integration Capabilities
The ability to integrate the annotation tool with other platforms and workflows is essential. Check if the tool can easily connect with your existing data management or machine learning frameworks.
Case Studies: Image Annotation in Action
1. Autonomous Vehicles
In the realm of autonomous driving, image annotation plays a crucial role in training models to recognize pedestrians, traffic signs, and other vehicles. Companies leverage techniques like bounding box annotations and segmentation to create rich datasets that improve vehicle navigation and safety.
2. Medical Imaging
Medical fields utilize image annotations to label anomalies in imaging data like X-rays and MRIs. Annotated images help in training diagnostic algorithms that can significantly enhance the efficiency and accuracy of medical diagnoses.
The Future of Image Annotation Tools in Machine Learning
The field of machine learning is ever-evolving, and so are the image annotation tools for machine learning. Emerging trends indicate a shift towards:
- Artificial Intelligence: Integration of AI to enhance the accuracy of automated annotation processes.
- Increased User Collaboration: Tools that promote collaboration across global teams, increasing diversity in data annotation.
- Real-Time Annotation: Tools optimizing real-time annotation to keep pace with fast-moving projects and datasets.
- Enhanced Visualization: Support for augmented reality and visualization features that provide deeper insights into annotated data.
Conclusion
As we have explored, image annotation tools for machine learning are indispensable for the successful implementation of machine learning projects. By ensuring high-quality data preparation through effective annotation practices, businesses can unlock powerful abilities in their applications. Whether you are in the automotive sector, healthcare, or any other industry leveraging visual data, investing in the right annotation tool can profoundly impact your success. Emphasizing accuracy, efficiency, and collaboration in your annotation process will pave the way for groundbreaking advancements in machine learning. Embrace these tools, and step into the future of data-driven decision-making.